Common Mistakes To Avoid When Venturing into AI
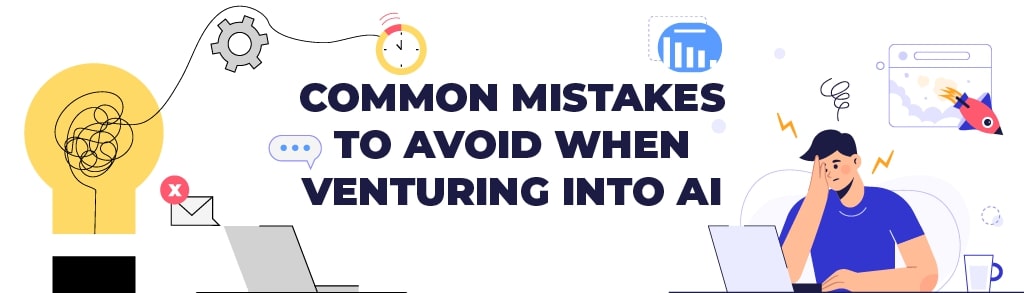
There’s no doubt about it– AI, or Artificial Intelligence, is all the rage in tech, and businesses are ready to cash in on the craze. In a recent IBM study, 3 out of 4 C-level executives predict that AI will play a “very important role” in the future of their organizations. And today, over 50 percent of the companies surveyed are already using AI in some capacity, often focused around automating marketing campaigns and accessing insights. And yet, while over 70% of executives surveyed believe that their industry is ready for AI, there are many mistakes happening along the way. The report indicates that many of these companies are not quite as ready as they think they are, and may be overestimating their capabilities. At 10Pearls, we see some key mistakes being made time and time again when a company adopts AI, and we navigate them at every turn. Look out for some of these as you begin your AI journey.
Assuming the project has an “end date”:
Implementing AI is not a project that begins and ends. In fact, AI modeling is very different from software development. Software is based on rules, often ones that are unchanging. Software turns data into output. Unlike software, an AI model is constantly changing, and works with what is probable, not what is certain. In order to build a successful AI platform, you must closely watch and evaluate it from the beginning of model design all the way through to its deployment and its evolution. Unlike software, AI keeps learning on its own, and changing itself.
So what does this mean? If AI is perpetually learning, and always changing, the teams that execute it need to be prepared to continue to adapt and evolve with the model. You need to know that this will be an ongoing process with value being realized at times exponentially and at times much more slowly, one that is not just directed by you, but by the underlying model, data and AI itself.
Forgetting to stand by your ethics:
AI and ethics– it’s a whole topic on its own. But it’s important to not just know what matters to you ethically, but to stick by it. Case in point: in that same IBM study, survey respondents listed their top three priorities for AI applications in 2021, and the number one answer was building “responsible AI tools to improve privacy, explainability, bias detection and governance”. It’s clear that they want to do the right thing, right? Except that when IBM asked them about their action plans for this year, around just ⅓ of respondents reported plans to actually improve its governance, reduce its bias, and ensure compliance with privacy regulations.
Ensure that your AI represents your values, or understand that if you don’t, that AI could quickly become totally incongruent with what you stand for. At 10Pearls, we create frameworks to continually assess current and planned AI models, checking for bias, as well as protection of privacy, amongst other things. We recommend you do the same.
Avoiding communication, up, down, and out:
One of the things that really surprised us when reading this study was that despite the fact that over half have already started working on AI, less than half of the respondents report having an AI strategy in place. That may stem from lack of broad strategy alignment and lack of understanding across the company leadership team.
Imagine that you are a VP of technology. You fully understand AI and its tremendous opportunities and implications, and you’re excited to carefully invest in this exciting category of tech. One need only watch the film “Coded Bias” or “The Social Dilemma” to show how the general public feels about AI impacting their lives. If you’re unable to explain this complex technology that many executives, including risk officers and even IT experts, don’t yet fully understand, you’ve got a problem.
Another interesting element of the IBM study was making AI more “explainable”. In the 2021 study, we actually see a dip in respondents focusing on explainability as opposed to the 2020 survey.
In order for your AI to be effective, there needs to be a strategy, the executives in your organization need to understand it at a high level, and you need to be able to defend it when questioned by anyone, including the public. Get your talking points in order before launching, and you’ll be in a much better position.
Hiring the wrong talent:
Of course, the most important choice you’ll make when developing AI is who you choose to build it. Whether hiring externally or internally, AI needs machine learning and model ops engineers with skills that blend software engineering with data science. Machine learning engineers help integrate, scale and deploy models, while Model ops engineers work on post deployment stability and monitoring. Good people in these roles are hard to find, and you need to select team members that you can trust. Look for companies that have a history of high performance in these areas, along with case studies that they can easily cite.
At 10Pearls, it’s our job to help you avoid making these mistakes when building technology.
Whether it’s helping you set a broad strategy for your AI efforts, creating a secure and ethical infrastructure upon which to build it, or delivering a high-quality brilliant team of engineers to get it done, we’ve got your back. It often starts with taking a product mindset where you set 90-day milestones to accomplish small steps. Experimentation and re-validation sets the basis for creating a framework that will evolve with time and help create future exponential value in your business. Click here to read more about how we can help.